4
APR 2019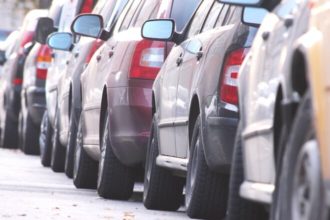
Case Update: Mileage Reimbursement
Posted by Carl McClain | Data Analytics, Employment, Wage and hour casesThe scope of Wage and Hour cases can extend beyond traditional claims on overtime or off-the-clock work. The same analytical principles can extend, for example, to cases involving employee reimbursements. EmployStats has recently worked on a case in California where the Plaintiffs allege they were not reimbursed for routine miles traveled in personal vehicles between job sites, despite the Defendant’s stated policy.
The EmployStats team assessed the Plantiffs’ theory of liability and estimated unreimbursed expenses based off of the available case data on mileage, parking, and toll charges. The analysis presented to the court showed a significant difference between stated and actual reimbursements for miles traveled by the Plantiffs. Based off of the analysis and other evidence at trial, the court certified the Plaintiff class.
The EmployStats Wage and Hour Consulting team’s trial plan is as follows:
- First, the EmployStats team would survey a statistically representative sample of class members about the existence of unreimbursed miles, using a random sampling methodology to eliminate potential bias.
- Next, the team would use a similar statistical sampling methodology to determine the typical miles traveled by the class members, and combining this resulting data with mapping platforms (ex. Google Maps API) to calculate distances in miles traveled between job locations.
- Finally, Employstats would tabulate damages based off of these results, using publicly available data on reimbursement rates for miles traveled in personal vehicles.