8
MAR 2019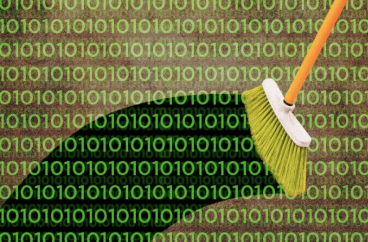
After acquiring and merging data, litigants will want to rush to an analysis. But raw datasets, no matter how perfectly constructed, are inevitably riddled with errors. Such errors can potentially bias or invalidate results. Data cleaning, the process which ensures a slice of data is correct, consistent, and usable, is a vital step for any data-based evidence.
There is a often quoted rule in data science which says 80% of one’s time is spent cleaning and manipulating data, while only 20% is spent actually analyzing it. Spelling mistakes, outliers, duplicates, extra spaces, missing values, the list of potential complications is near infinite. Corrections should be recorded at every stage, ideally in scripts of the program being used (ex. R, SAS, SQL, STATA); data cleaning scripts leave behind a structured, defensible record. Different types of data will require different types of cleaning, but a structured approach will produce error free analytical results.
One should start with simple observations. Look at batches of random rows, what values are stored for a given variable, and are these values consistent? Some rows may format phone numbers differently, inconsistently capitalize, or round values. How many values are null, and are there patterns in null entries? Calculate summary statistics for variables, are there obvious mistakes (ex. negative time values)? After an assessment, cleaning can begin.
Fixing structural errors is straightforward: input values with particular spellings, capitalization, split values (ex. data containing ‘N/A’ and ‘Not Available’), or formatting issues (ex. numbers stored as strings rather than integers) can be systematically reformatted. Duplicate observations, common when datasets are merged, can be easily removed.
However, data cleaning is not entirely objective. Reasonable assumptions must be made when handling irrelevant observations, outliers, and missing values. If class X or transaction type Y is excluded from litigation, its reasonable to remove their observations. However, one cannot automatically assume Z, a similar class, can be removed as well. Outliers function the same way. What legal reasoning do I have to remove this value from my dataset? Suspicious measurements are a good excuse; but, just because a value is too big or too small, that alone does not make it reasonable to remove.
Missing data is a difficult problem: how many missing or null values are acceptable for this analysis to still produce robust results? Should you ignore missing values, or should you generate values based off of similar data points? There is no easy answer. Both approaches assume missing observations are similar to the rest of the dataset. But the fact that the observations are missing data is informative in of itself. A more cautious stance, the one with the least assumptions, will inevitably be easier to defend in court.
Skipping data cleaning, and assuming perfect data, casts doubt on any final product. Data-based evidence follows the maxim “garbage in, garbage out.”