7
JAN 2022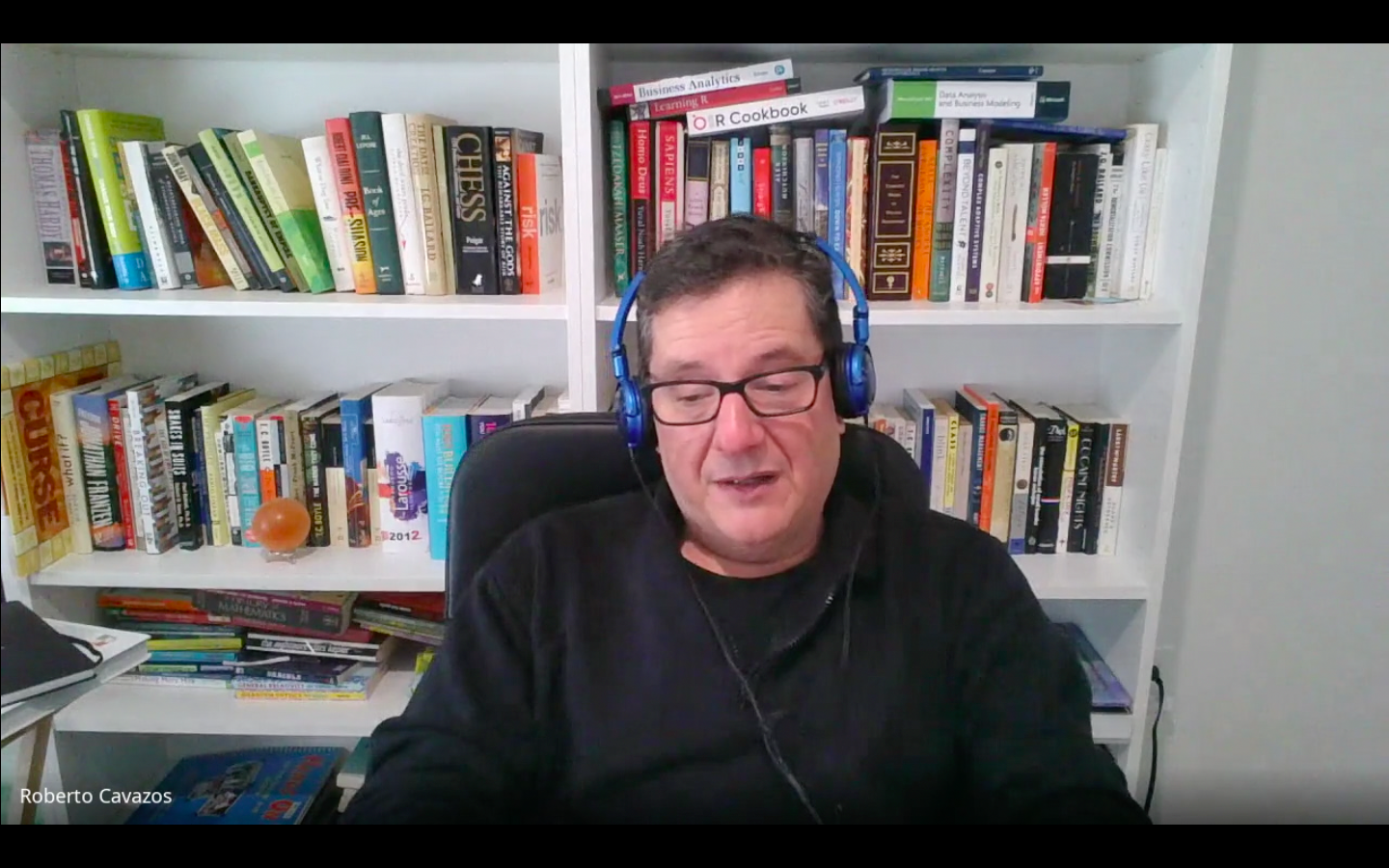
Dr. Roberto Cavazos Discussing the Economic Impact of Pay-Per-click Fraud
Posted by Emma Dooley | Data Analytics, Economics, Industry, U.S. Economy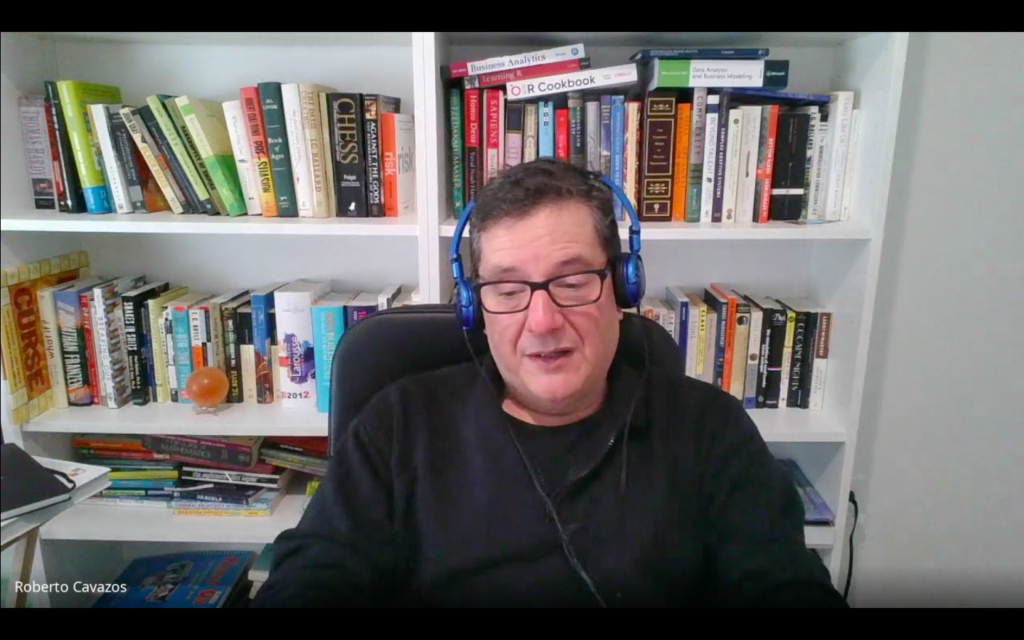
EmployStats economist Dr. Roberto Cavazos finds that economic loss from pay-per-click (PPC) is on the rise as fraudulent activity risks have increased as many large corporations use this platform. According to Dr. Cavazos, approximately $144 Billion is spent on paid and social search globally. However, according to empirical-based research, 14% of PPC was fraudulent making the economic loss to $23.7 Billion by the end of 2020.
What exactly is PPC fraud? According to bigcommercec.com, PPC fraud can be defined as individuals, computer programs, or generated scripts exploiting online advertisers by repeatedly clicking on a PPC advertisement to generate fraudulent charges. Dr. Cavazos stated there are several techniques used to perform these acts including competitor clicks, clicks by automated clicking tools, and robots or other deceptive software. To counteract these invalid clicks, Google is taking a proactive approach by automatically filtering any click that is considered invalid, however many clients find this safeguard to be a lot more complicated than beneficial.
Dr. Cavazos reported the economic loss that has amounted from PPC globally has made a substantial dent in the market. The total PPC fraud loss for the U.S. in 2019 was $7,700,000,000 and $9,060,800,000 in 2020. By the end of 2020, the expected loss for the largest eCommerce sector was $3.8 Billion on PPC campaigns. This was based on the 17% fraudulent clicks that were found across multiple eCommerce campaigns. Dr. Cavazos shared even though global web sales amounted to an astounding $3.4 trillion in 2019, the projected loss from PPC fraud is still a sizeable amount. Despite the global loss, paid search remains the most-used digital advertising format bringing in 47% of total digital revenues.
The rise of PPC platform spending has opened a new route for commitment of fraudulent activity. Each platform experiences their own issues with fraudulent activity making it difficult to recognize. Ending PPC fraud has become a top priority when it comes to enhancing and augmenting future campaigns. While PPC has been a successful marketing tactic, it poses risks for fraudulent behavior requiring an understanding of the consequence of the use of PPC and steps to be taken to minimize those risks. Dr. Cavazos believes these losses are borne by businesses making the smaller ones most vulnerable to suffering PPC fraud.